The term AI can mean many different things. Some describe it as a suitcase term— everyone uses it differently, and there is a lot to unpack.
If CEOs, leaders, and investors understand what people mean when talking about AI, they will communicate more clearly, make better decisions, and appropriately respond to concerns.
For example, with the instant popularity of ChatGPT, many people use AI to mean only that. You will miss many opportunities if your AI conversations start and end with ChatGPT.
This essay is my attempt to clarify how people use the term1. Even though you can clearly define AI within your organization, you cannot control how the term is used by peers, other companies, vendors, the media, board members, and likely even your employees.
To unpack the term, we need a little history.2
1956 to ~2000: The rise of the term AI
In 1956, top researchers gathered at Dartmouth to create machines that could replicate human intelligence. They coined the term AI for this work. Today, we would call this Artificial General Intelligence (AGI), but more on that later.
Periodically, breakthroughs would capture the public’s imagination and make it seem like we were on the brink of machines having human intelligence. But each time, people would become disillusioned when the reality did not live up to the hype.
There was a name for these down periods: AI Winters. During an AI winter, people would shy away from working on AI, and funding would dry up.
By the early 2000s, AI wasn’t used much. Here is where we were:
First, some of the early AI research that wouldn’t work for general intelligence turned out to help solve industry problems, like expert systems.
Second, people knew what the term AI meant. At this point, it was mostly for science fiction. The Terminator movies, for example, kept the term alive in popular culture.
Third, CEOs, leaders, and investors weren’t thinking about AI. They had been thinking about Y2K and then the internet. No one talked about AI as a serious tool for businesses and organizations.
2006: The Rise of Analytics
The analytics movement plays a big role in how people use the term “AI” today. At the time, no one used AI to describe this work.
In 2006, Tom Davenport published a Harvard Business Review article, “Competing on Analytics.3” Many people credit this with kicking off the analytics movement.
Of course, before 2006, plenty of organizations had already been using data, statistics, simulation, optimization, and a host of other sophisticated algorithms to solve problems.
The time was ripe for the movement to start. Companies had more data than ever from their ERP systems, websites, and sensors. They wanted to use the data for better insights and decisions.
I heard an interview with Tom Davenport. He was asked why his article struck such a nerve. His answer, if I recall, was that he was a bit lucky with the title. The title scared CEOs, leaders, and investors: They worried they would fall behind if they didn’t use data and analytics.
The term analytics (and the related term Big Data) went viral. In addition, machine learning algorithms were rediscovered and created excitement.
Like AI, “analytics” was also a suitcase term. From my view, the most common misconception about analytics was that it was just about reporting. For other types of analytics, we had to say something like “advanced analytics.”
Luckily, a framework emerged that defined the three types of analytics:4
Descriptive Analytics: (What happened). This is analyzing historical data to find insights through better reports or dashboards.
Predictive Analytics (What will happen). This applies algorithms to data to predict what will happen or classify data better. Most machine learning algorithms fit here.
Prescriptive Analytics (What should we do). This is applying algorithms to the data to help make better decisions. Optimization is the primary technology in this area.
By 2015-2016, the analytics movement was rolling. It delivered value in various ways for all kinds of industries. Organizations were talking about analytics, building teams, and getting things done.
The analytics movement was about to combine with the AI movement.
2012: The world was given notice that it was AI Spring again.
While the analytics movement was rolling along, AI researchers continued to work on the 1956 vision. In the early 2000s, the primary focus was on neural networks (especially deep neural networks, also called deep learning).
A neural network is an algorithm modeled after our understanding of how the brain works.
The big breakthrough of 2012 came in an image recognition contest. The contest was to see what algorithm could best recognize images. In 2011, a standard machine learning algorithm won the contest.
In 2012, three things came together: robust neural network algorithms, widely available image data from the internet, and numerous GPU processors.
In 2012, the neural network won by a wide margin and was comparable to what a person could do.
Since recognizing images seems like something human-like and neural network algorithms work like the brain, it was only natural that the media picked up on the term AI.
Money poured into neural networks for image and video recognition, translation, voice-to-text, and playing games. Self-driving cars didn’t take long to get added to the list.
2022: ChatGPT
ChatGPT was released in late 2022. This was an extension of the rush that started in 2012. It is worth pointing out because it made such a big splash. The 2012 breakthrough was big news, but it took a while to get moving and was confined to a relatively small part of the overall economy and hardly touched consumers.
With ChatGPT5 and the drawing applications (like DALL-E) that came earlier, ordinary people could see the power and magic of these algorithms.
The terms AI and analytics seemed popular before ChatGPT. I didn’t realize how much ChatGPT would change the conversation: everyone was talking about AI.
Enough History: Let’s pull it all together with four definitions of AI and actions for each
As you can see from above, there are a lot of different pieces: the goals of the 1956 meeting, the analytics movement, advances in deep learning, and ChatGPT.
Given all of this, here are the four ways I see people use the term AI, each with opportunities or warnings.
Definition #1: When people say AI, they can mean the same thing they meant in 1956: creating machines with human-like intelligence. This is also called AGI- Artificial General Intelligence.
The “G” in AGI is often added because of the definitions you’ll see later. Sometimes those other areas are called “Narrow AI” because they only solve a specific problem.
This definition is the most faithful to the work started in 1956.
AGIT is also called superintelligence and “the singularity” (when machines get smarter than humans and can take over intelligent activity on Earth).
It should be pointed out that we have yet to achieve AGI. With the release of ChatGPT, many people thought we were much closer. Or, we may still be very far away. There have been many such claims before that have turned into dead-ends for AGI. AGI is still an active area of research.
Unless your organization is doing specific AGI research, the main reasons to follow this area are to address the fears of AGI and watch for work that can have practical applications.
If people fear the end of civilization from AI, they are thinking of AGI. (Here is another post with more information on that.)
As far as practical applications, many come out of AGI research. For example, even if deep learning and ChatGPT don’t lead to AGI, they will still be two of our greatest inventions with many valuable applications.
Definition #2: When people say AI, they can mean using deep learning to solve hard problems.
The second definition comes from the fact that deep learning algorithms have driven the recent resurgence in AGI research. Therefore, by extension, some people call all deep learning algorithms AI. And, by extension, anything not using deep learning is not AI.
For example, instead of saying that deep learning is the technology behind self-driving cars, AlphaFold, and victories in the ancient game of Go, people say that it is AI. (ChatGPT will also get lumped in here, but more on that next.)
There is nothing wrong with using AI like this. However, this definition has two lessons for CEOs, leaders, and investors.
The first is the stretch lesson: are there tough problems that deep learning could solve that would change your industry or organization?
The second is the warning lesson: if you think that AI has to be just deep learning, you will miss many opportunities (see definition #4) and may overengineer many solutions (that is, your team builds a complex and data-hungry solution when a much simpler algorithm would have done the trick).
Definition #3: When people say AI, they can mean ChatGPT (and similar tools). This is also called Generative AI.
Since ChatGPT touched so many people and was the first direct exposure people had to the power of deep learning algorithms, many people now equate AI with only these tools.
Of course, like above, the danger with this definition of AI is that you limit the power of AI by not considering deep learning for hard problems (like definition #2) or not considering the range of other valuable algorithms (like definition #4).
On the other hand, people are right to recognize the power of ChatGPT to change how we work.
No one knows how this technology will shake out. However, as a starting point, it is helping with writing, summarizing coding, learning (a personal tutor), brainstorming, and many more task. Organizations are already working to create natural language interfaces for customer service or their products. You may want a workstream investigating how this technology will impact your organization.
There is a continuous stream of interesting new ideas with ChatGPT. You could build a career helping your organization best use this technology.
Definition #4: When people say AI, they can also include what used to be called predictive and prescriptive analytics. This might also be called Practical AI or Enterprise AI.
As mentioned above, forgetting all the valuable ideas from the analytics movement would be a mistake. That is, there are a host of valuable algorithms that aren’t deep learning or ChatGPT. These algorithms should play a significant role in your AI strategy.
Many already recognize this. This is why much of the predictive and prescriptive analytics work was rebranded as AI around 20176.
You can see this from the chart below showing the big increase in companies mentioning AI by 2017.
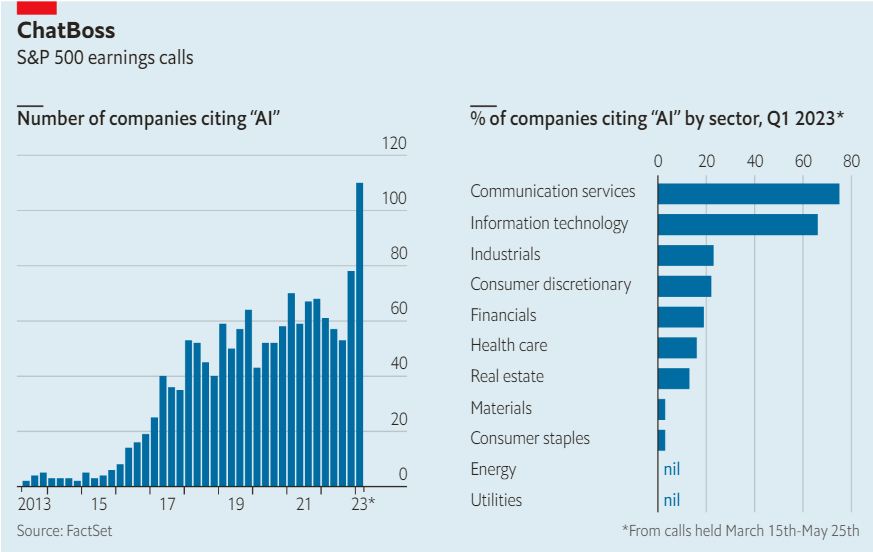
In 2017, ChatGPT was far away, and deep learning was still gaining momentum. People were already rebranding analytics as AI. I would like to think that the more serious thinkers still used the term analytics for descriptive analytics.
The positive reason for calling this AI is that it brings attention to this type of work. And, in fairness to many of these algorithms, they can be said to solve hard problems that human intelligence cannot match. For example, mathematical optimization can schedule workforces, route trucks, set inventory, allocate energy, and do various other tasks unmatched by any human (or by any deep learning or ChatGPT algorithm).
In addition, the analytics movement has given us time to develop processes for bringing value to your organization, decreasing the risk. The following two books are my top recommendations for organizations wanting to implement Practical AI.
The downside of calling this work AI is that you can fool yourself into thinking you are doing AI when you use only a few sophisticated algorithms. You don’t want to use this definition to fool yourself into thinking you have an AI organization.
Wrap Up
If I had to summarize the opportunity for CEOs, leaders, and investors in the four definitions above, I would say:
Understand AGI enough to have talking points and a position on potential threats.
Look for opportunities for deep learning to solve the hardest problems.
Figure out how ChatGPT will change your organization.
Don’t forget the potential opportunity with Practical AI (prescriptive and predictive analytics).
I’ll finish quotes from Tyler Cowen, who was mostly talking about ChatGPT (my emphasis):
“It will disrupt many of us, often in good ways, sometimes in bad ways…But I would just here urge against complacency… …It will be a fascinating future. Very weird in many ways…but we should all be ready for it.”7
Subscribe for free and get the latest posts in your e-mail
This essay builds on my previous writings but is meant to be standalone.
This essay was inspired by Melanie Mitchell’s book Artificial Intelligence. She covers the history of AGI quite well. Here is my review of her book.
A year later, he published a book with the same title, co-authored with Jeanne Harris
Some frameworks had other categories, like diagnostic analytics. But these three were the most popular.
For simplicity, and I know it isn’t fair, I will refer to this class of Large Language Models as ChatGPT. I realize that there are plenty of other competitors and new approaches. When I talk about ChatGPT, include all these other similar solutions too.
This wasn’t a conscious decision; it just emerged. I think the AI sounded cooler and was easier to say.
From Econtalk interview on May 15, 2023.
Mike, excellent write-up! I have a quick question. I keep coming across the term diagnostic analytics (the why something happened?). I was curious as your thought about it being a 4th type of analytics.